Biomedical signal processing involves acquiring and preprocessing physiological signals and extracting meaningful information to identify patterns and trends within the signals.
生物医学信号的来源包括神经活动,心脏节律,肌肉运动和其他生理活动。诸如心电图(ECG),脑电图(EEG),肌科(EMG)的信号可以非侵入性地捕获并用于诊断和整体健康的指标。
The biomedical signal processing workflow involves:
- Signal Acquisition
- Signal Visualization and Annotation
- Artifact Removal and Preprocessing
- Feature Extraction
The extracted features are then fed into classification models or used directly for diagnosis.

Workflow for processing biomedical signals.
MATLAB®为此工作流提供许多信号处理能力,尤其是用于信号预处理和特征提取。
Signal Acquisition:With MATLAB, you can interface with hardware equipment to acquire physiological signals. For instance, with the Raspberry Pi and Arduino Support Packages, you can interface with embedded boards like Raspberry Pi, Arduino, and EKGShield to collect data from these sensors. You can also access and analyze signals stored in files such as from EDF, Excel®文件和mat文件。
Signal Visualization and Annotation:MATLAB提供内置应用程序,以帮助您分析和可视化时间,频率和时频域中的信号,而无需写入任何代码。这些功能可以帮助您了解您可以使用的预处理技术来增强信号中包含的信息。
You can also annotate the biomedical signals and prepare them for downstream workflows such as machine learning and deep learning using the Signal Labeler app. Signals can be labeled manually or using algorithms like those that find peaks and transition points.

Analyzing an ECG signal in time, frequency and time-frequency domains with the Signal Analyzer app in MATLAB.
伪影删除和信号过滤:Biomedical signals often contains noise or unwanted artifacts that can distort the analysis of the signals. For instance, while measuring EKG signals, activities such as breathing and walking can add unwanted components. One of the main challenges in preprocessing biomedical signals is to remove unwanted artifacts while preserving the sharp features within signals. Most popular techniques for artifact removal are digital filtering, adaptive filtering, independent component analysis (ICA), and recursive least square. A combination of preprocessing techniques may also be used to address the limitations of individual techniques.

Digital filter used for signal preprocessing.
Feature Extraction with Signal Processing:Feature extraction can be accomplished manually or automatically. Signal processing techniques like AR modeling, Fourier analysis, and spectral estimation can be used to manually compute key features from signals. Time-frequency transformations, such as the short-time Fourier transform (STFT) can be used as signal representations for training data in machine learning and deep learning models. Automatic feature extraction techniques like wavelet scattering can be used to reduce dimensionality and extract important features. These features can be used directly for diagnosis or as input to machine learning and deep learning classifiers.
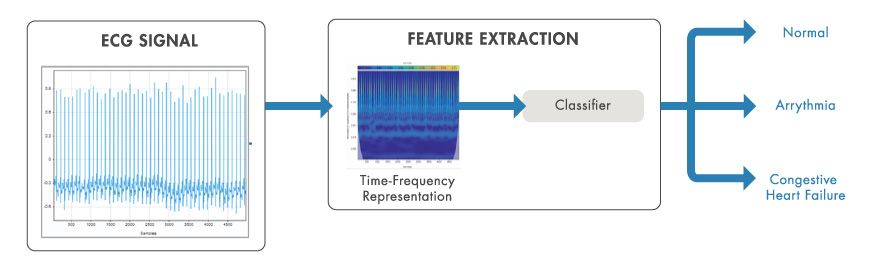
时间频率分析用于从ECG信号中提取分类的特征。
在开发信号处理算法后,您可以通过自动生成实时代码来创建概念和概念的原型设计。您还可以生成VHDL和Verilog代码,以在FPGA和ASIC等设备上部署信号处理算法,以便原型和构建低功率医疗设备。MATLAB Coder™和Embedded Coder™允许您生成优化的C / C ++代码以在嵌入式硬件上部署算法。高性能GPU可用于加速预处理,特征提取和模型推断。
For more details, seeSignal Processing Toolbox™和Wavelet Toolbox™